Betaworks is embracing the AI trend not with yet another LLM, but instead with a series of agent-like models that automate everyday tasks that aren’t so easy to define. The investor’s latest incubator “Camp” trained and funded nine agent AI startups that they hope will take on today’s most tedious tasks.
The use cases for many of these companies sound promising, but AI tends to struggle to deliver on its promises. Would you trust a shiny new AI to sort your email for you? What about extracting and structuring information from a web page? Would anyone be interested in AI slot meetings wherever it works?
There is an element of trust that has yet to be established with these services, which is the case with most technologies that change the way we act. Asking MapQuest for directions was weird until it didn’t — and now GPS navigation is an everyday tool. But are AI agents at that stage? Betaworks CEO and founder John Borthwick believes so. (Disclosure: Former TechCrunch editor and Disrupt host Jordan Crook has left TC to work at the company.)
“You’re moving on to something that we’ve spent a lot of time thinking about,” he told TechCrunch. “While agent AI is in its infancy – and there are issues around agent success rates and so on – we’re seeing huge strides even since Camp started.”
While the technology will continue to improve, Borthwick explained that some customers are ready to embrace it in its current state.
“Historically, we’ve seen customers take a leap of faith, even with higher stakes jobs, if a product was ‘good enough.’ The original Bill.com, despite doing interesting things with OCR and email scraping, didn’t always get it right, and users still trusted it with thousands of dollars worth of transactions because it made a terrible job less terrible. And over time, through highly communicative interface design, feedback loops from those customers have created an even better, more reliable product,” he said.
“Currently, most of the early adopters of the products at Camp are developers and founders and early adopters of technologies, and this team has always been willing to patiently test and provide feedback on these products, which eventually jump into the mainstream” .
Betaworks Camp is a three-month accelerator where select companies in their chosen topic get hands-on help with their product, strategy and connections before being kicked out the door with a $500,000 check — courtesy of Betaworks itself, Mozilla Ventures , of Differential Ventures and Executive AI. But not before the startups strut their stuff on demo day on May 7.
However, we did take a look at the lineup beforehand. Here are the three that stood out to me the most.
Twin automates tasks using an “action model” that we’ve been hearing Rabbit talk about for a few months now (but they haven’t shipped yet). By training a model on lots of data representing software interfaces, it can (these companies argue) learn how to complete common tasks, things that are more complex than an API can handle, but not so much that they can’t be assigned to a “smart intern.” We actually wrote them in January.
Image Credits: Twin
So instead of having a back-end engineer create a custom script to perform a specific task, you can demonstrate or describe it in plain language. Things like “put all the resumes we received today into a Dropbox folder and rename it to the applicant’s name, then send me the share link on Slack.” And after tweaking that workflow (“Oops, this time add the implementation date to the filenames”) it might just be the new way the process works. Automating the 20% of tasks that take up 80% of our time is the company’s goal — whether it can do it economically is perhaps the real question. (Twin declined to elaborate on the nature of the model and the training process.)
Skej aims to improve the sometimes arduous process of finding a meeting time that works for two (or three, or four…) people. Simply populate the bot in an email or Slack thread and it will begin the process of reconciling everyone’s availability and preferences. If he has access to schedules, he will check them. If someone says they’d prefer the afternoon if it’s Thursday, work with that. You can tell some people have priority. and so on. Anyone who works with a dedicated executive assistant knows they’re irreplaceable, but chances are every EA out there would rather spend less time on tasks that are just a bunch of “What do you say? No? How about that?”
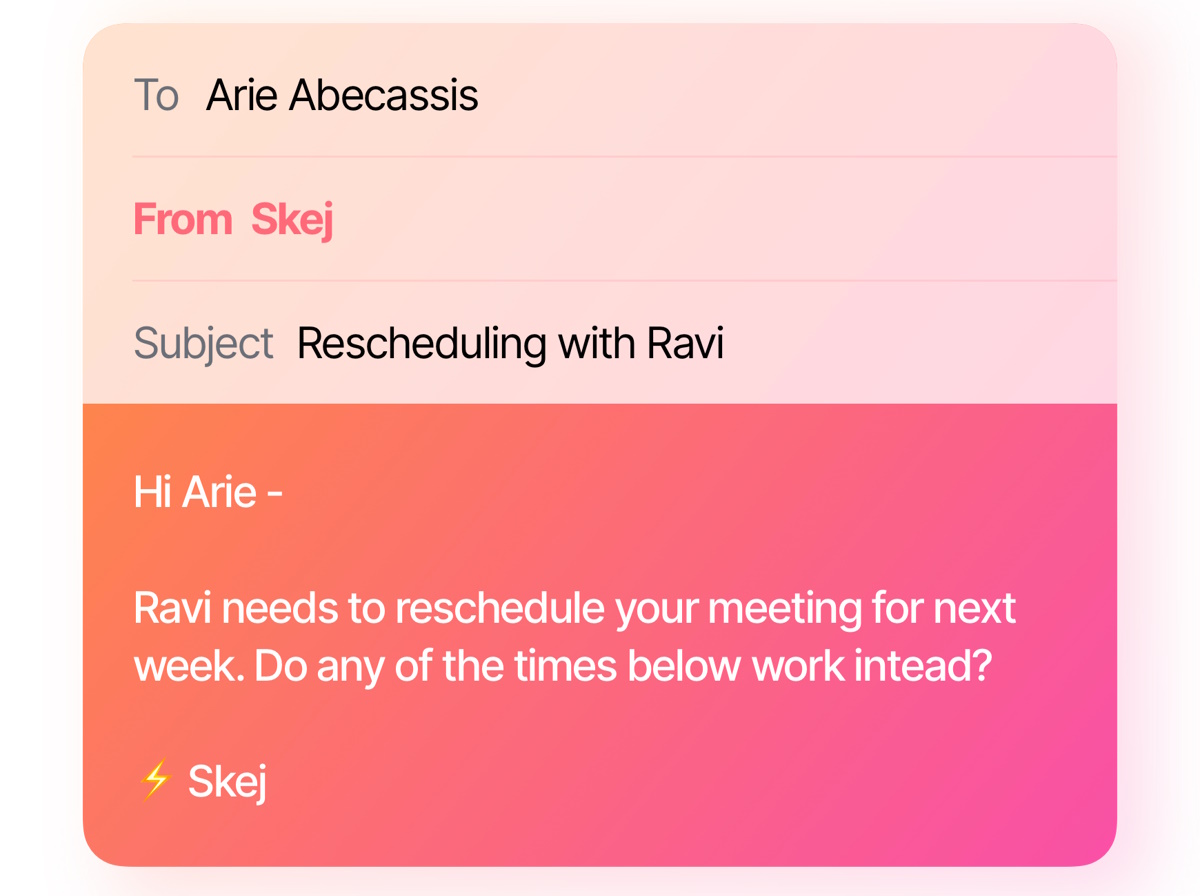
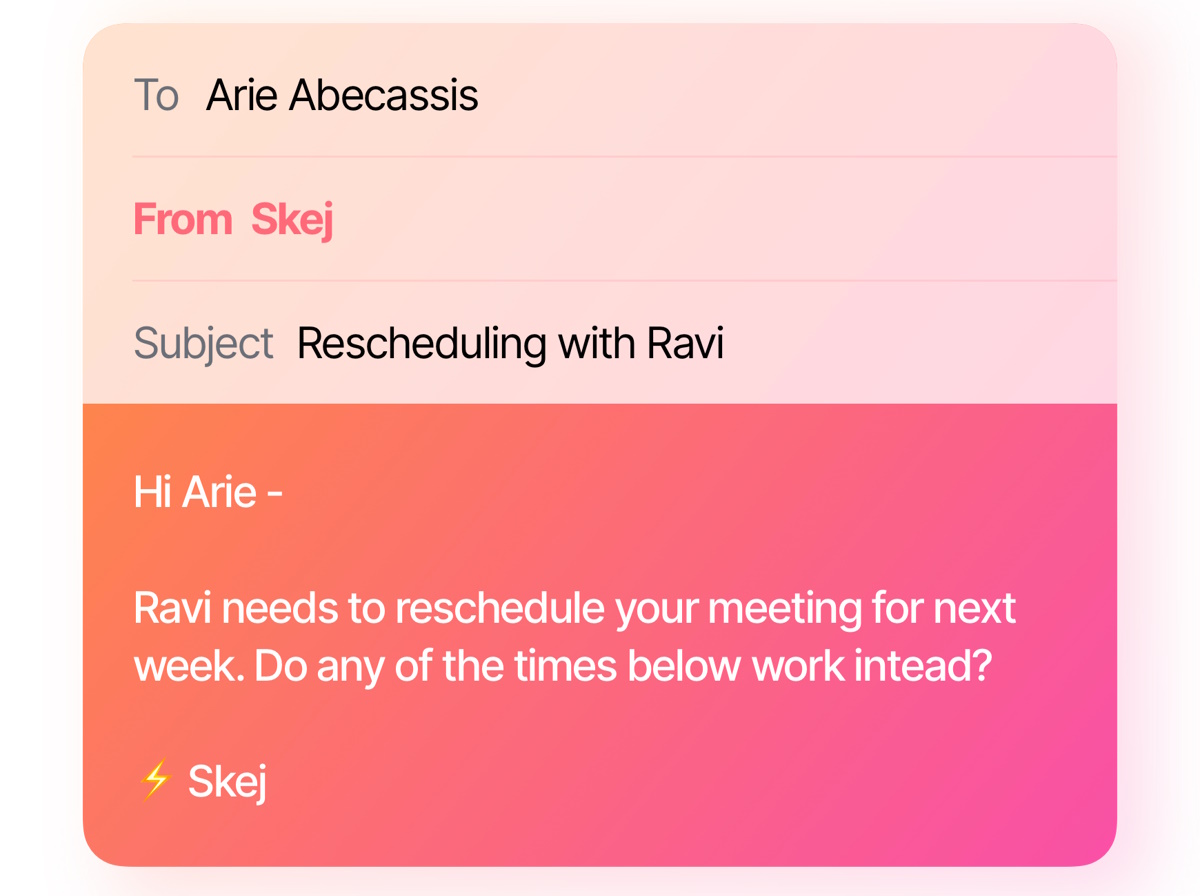
Image Credits: Skej
As a misanthrope, I don’t have this programming problem, but I appreciate that others do, and I’d also prefer a set-it-and-forget-it solution where they just accept the results. And it’s well within the capabilities of today’s AI agents, who will be primarily tasked with understanding natural language rather than patterns.
Jsonify is an evolution of web scrapers that can extract data from relatively unstructured environments. This has been done for ages, but the engine that extracts the information has never been so clever. If it’s a big, flat document, they work fine — if it’s in in-place tabs or some poorly coded visual list meant for people to click, they can fail. Jsonify uses the improved understanding of today’s visual AI models to better analyze and classify data that may not be accessible to simple crawlers.


Image Credits: Jsonify
So you could do a search for Airbnb options in a given area and then have Jsonify put them all into a structured list with columns for price, distance from airport, rating, hidden fees, etc. . Then you can do the same thing in Vacasa and export the same data — maybe for the same places (I did this and saved $150 the other day, but I wish I could have automated the process). Or, you know, do professional things.
But doesn’t the imprecision inherent in LLMs make them a questionable tool for the job? “We’ve been able to build a pretty robust guardrail and cross-check system,” said founder Paul Hunkin. “We use a few different models at runtime to understand the page, which provide some validation — and the LLMs we use are tailored to our use case, so they’re usually pretty reliable even without the protective layer. We typically see 95%+ extraction accuracy, depending on the use case.”
I could see any of this being useful in probably any technology promotion business. The others in the cohort are a bit more technical or casual — here are the remaining six:
- AI solving – agent automation of cloud workflows. It feels useful until the custom integrations cover it.
- Flood – an artificial inbox scrambler that reads your email and finds the important stuff while preparing appropriate responses and actions.
- Scalable AI – is your AI lagging? Ask your doctor if Extensible is the right background screening and recording for your development.
- Opponent – a virtual character intended for children to have extensive interactions with and play with. It feels like a minefield morally and legally, but one has to walk it.
- High dimensional research – the infrared game. A framework for web-based AI agents with a payment model, so if your company’s experiment craters, you’re only owed a few bucks.
- Body – Generative AI for robotics, a field where training data is comparatively scarce. I thought it was an Afrikaans word, but it’s just ’embody’.
There is no doubt that AI agents will play a role in the increasingly automated software workflows of the near future, but the nature and extent of that role has yet to be written. It’s clear that Betaworks is aiming to get its foot in the door early even if some of the products aren’t yet ready for their mass market debut.
You will be able to see the companies showing off their representative products on May 7th.
Correction: This story has been updated to reflect that the founder of Jsonify is Paul Hunkin, not Ananth Manivannan.